Introduction #
Authors introduce the Branch component for instance segmentation within The Tree Dataset of Urban Street, encompassing 1,485 high-resolution images distributed across 13 distinct classes (branch_of_albizia_julibrissin, branch_of_flowering_cherry etc.). This specific section is designed to facilitate the precise delineation and identification of individual tree instances within the urban landscape. With these comprehensive resources at your disposal, this subset empowers researchers and practitioners to delve deep into the detailed analysis of urban street greenery, offering a valuable resource for comprehensive instance segmentation studies. Automatic tree species identification can be used to realize autonomous street tree inventories and help people without botanical knowledge and experience to better understand the diversity and regionalization of different urban landscapes.
The Tree Dataset of Urban Street sub-datasets:
Classification:
- Branch 1485 images, 13 classes (1.4G) (current dataset includes classification tags)
- Trunk 7675 images, 29 classes (6.4G) (available on DatasetNinja)
- Leaf 21127 images, 50 classes (13.6G) (available on DatasetNinja)
- Tree 4804 images, 23 classes (4.3G) (available on DatasetNinja)
- Fruit 4101 images, 29 classes (2.1G) (available on DatasetNinja)
- Flower 2275 images, 17 classes (1.3G) (available on DatasetNinja)
Segmentation:
- Tree 3949 images, 22 classes (7.9G) (available on DatasetNinja)
- Branch 1485 images, 13 classes (3.1G) (current)
- Trunk 7675 images, 29 classes (12.9G) (available on DatasetNinja)
- Leaf 9763 images, 39 classes (10.2G) (available on DatasetNinja)
Detection:
- Leaf 9763 images, 39 classes (11G) (available on DatasetNinja)
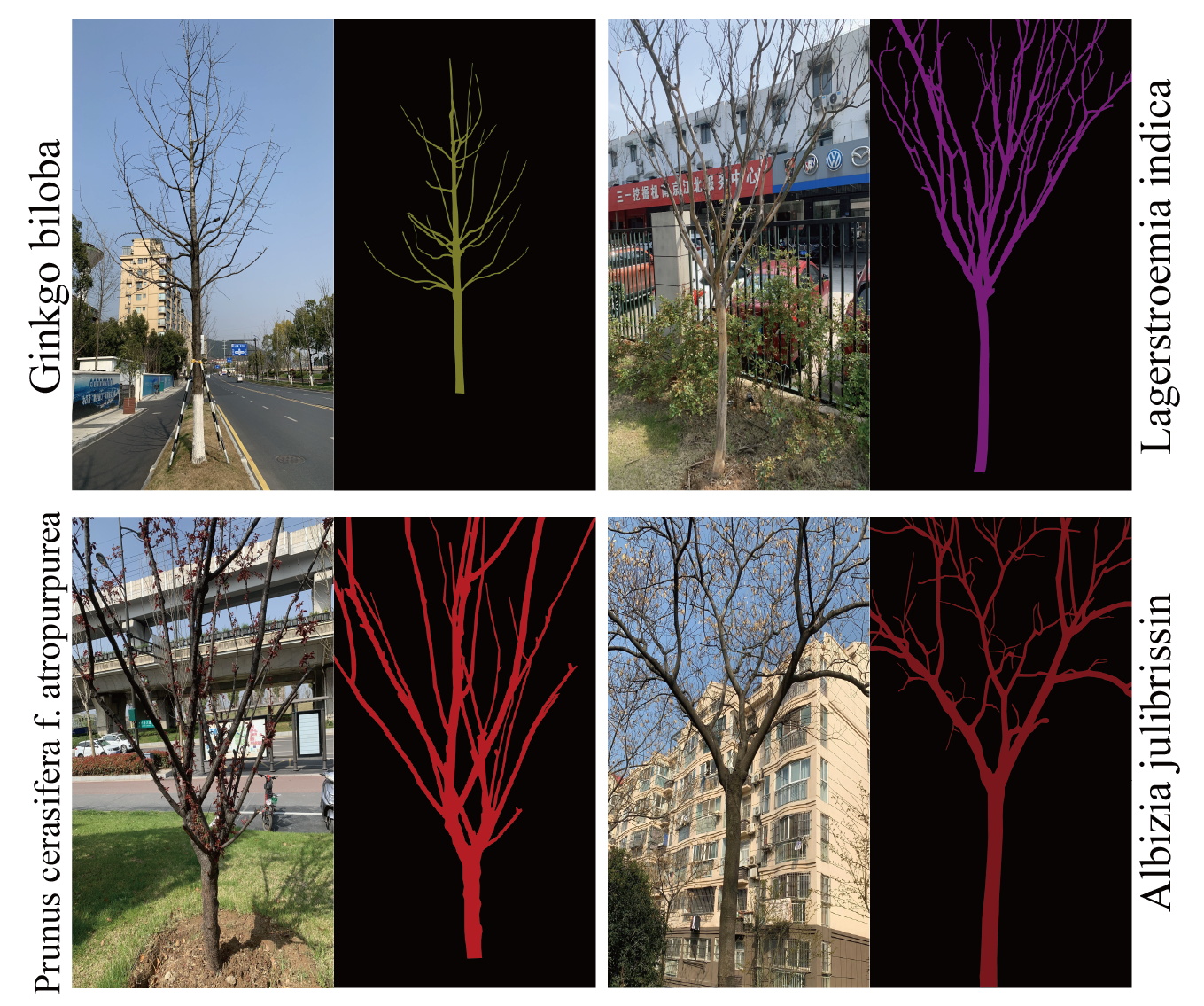
Examples of Urban Street: Branch (segmenation task).
About Tree Dataset of Urban Street
Annotations were performed in a fine-grained manner by using polygons (bitmap in supervisely) to outline individual objects. Authors assessed the performance of various vision algorithms on different classification and segmentation tasks, including tree species identification and instance segmentation.
The proposed dataset was designed to capture urban street trees with subtropical or temperate monsoon climates in China. Our data collection and annotation methods were carefully created to capture the high variability of street trees. From February to October 2022, tens of thousands of tree images were acquired with mobile devices, covering spring, summer, fall and winter in 10 cities.
Similar to Cityscapes (Cordts et al., 2016) (available on DatasetNinja) and ADE20K (Zhou et al., 2019) (available on DatasetNinja), authors divide each organ dataset into separate training (train), validation (val) and test (test) sets.
Summary #
Tree Dataset of Urban Street: Branch is a dataset for instance segmentation, semantic segmentation, object detection, and classification tasks. It is used in the environmental industry.
The dataset consists of 1485 images with 1556 labeled objects belonging to 13 different classes including branch_of_flowering_cherry, branch_of_lagerstroemia_indica, branch_of_prunus_cerasifera_f._atropurpurea, and other: branch_of_liriodendron_chinense, branch_of_zelkova_serrata, branch_of_magnolia_liliflora_desr, branch_of_styphnolobium_japonicum, branch_of_triadica_sebifera, branch_of_ginkgo_biloba, branch_of_koelreuteria_paniculata, branch_of_salix_babylonica, branch_of_platanus, and branch_of_albizia_julibrissin.
Images in the Urban Street: Branch dataset have pixel-level instance segmentation annotations. Due to the nature of the instance segmentation task, it can be automatically transformed into a semantic segmentation (only one mask for every class) or object detection (bounding boxes for every object) tasks. All images are labeled (i.e. with annotations). There are 3 splits in the dataset: train (1193 images), val (149 images), and test (143 images). Also, the dataset includes classification_tag. The dataset was released in 2022 by the Zhejiang Agriculture and Forestry University.
Here are the visualized examples for the classes:
Explore #
Urban Street: Branch dataset has 1485 images. Click on one of the examples below or open "Explore" tool anytime you need to view dataset images with annotations. This tool has extended visualization capabilities like zoom, translation, objects table, custom filters and more. Hover the mouse over the images to hide or show annotations.
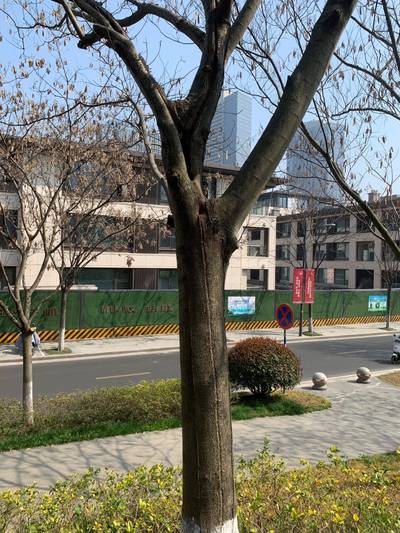

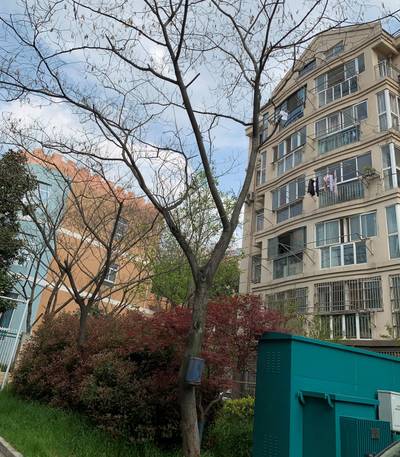
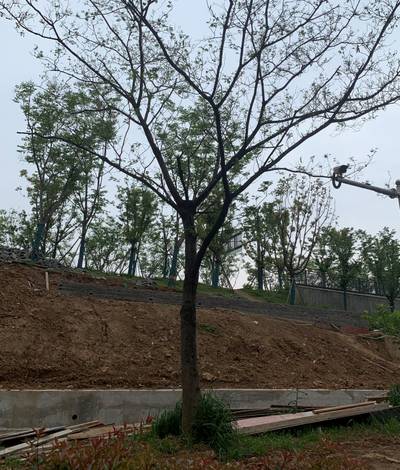


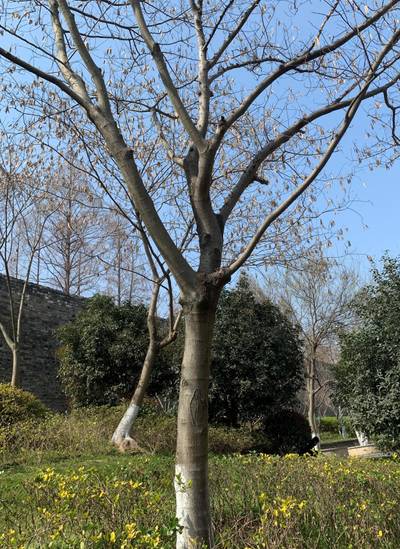
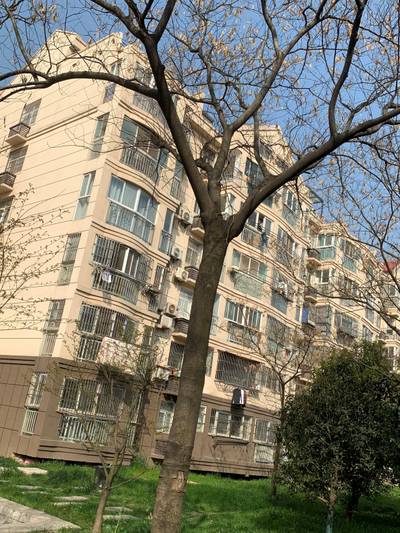


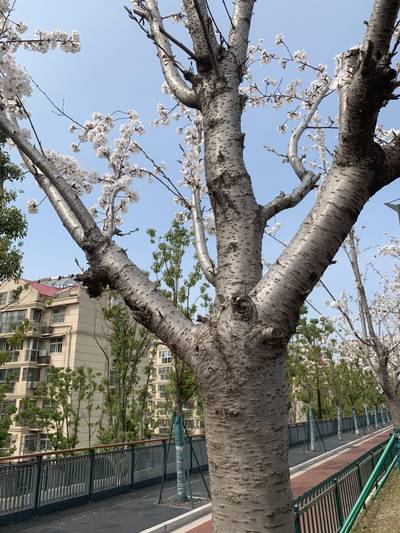
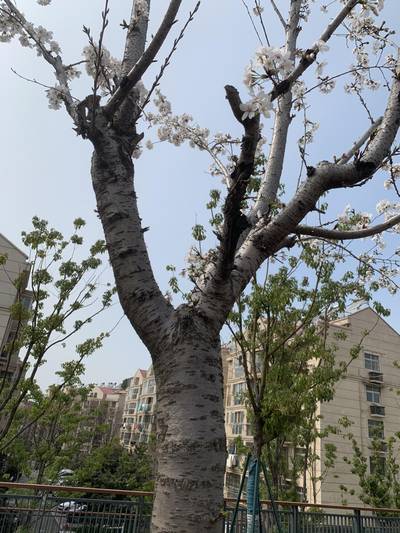
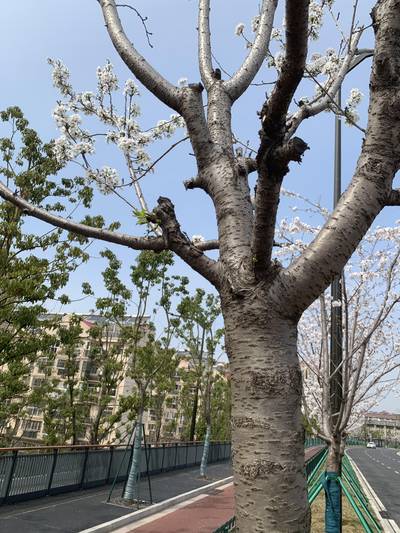
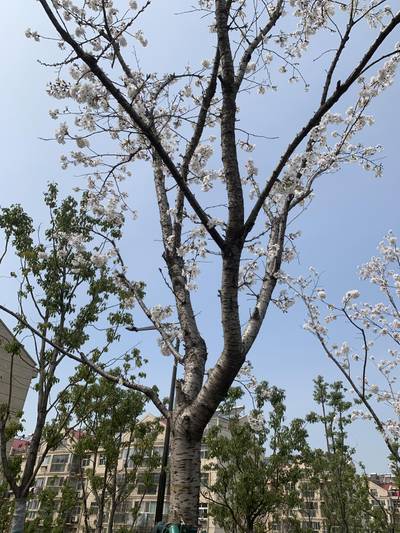
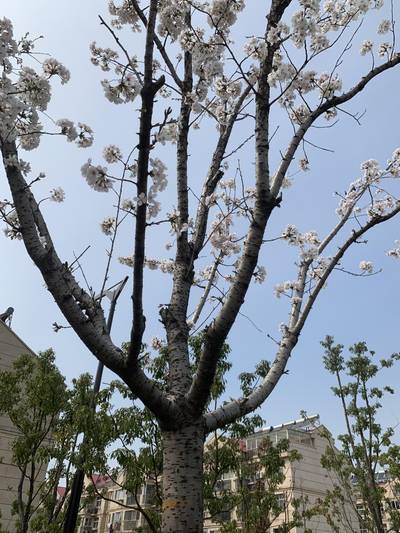

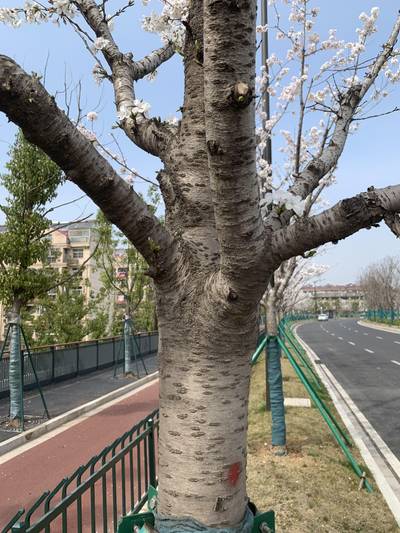
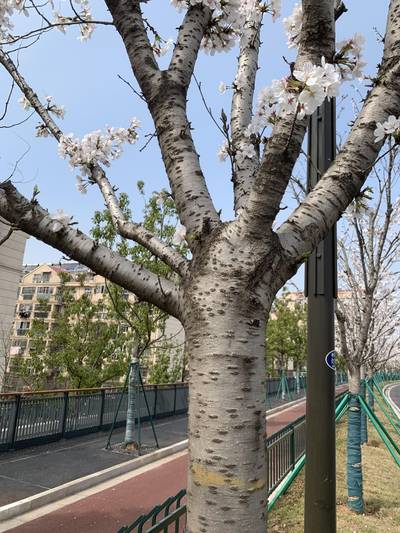
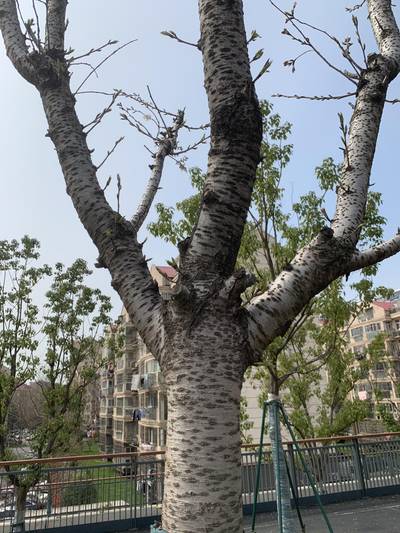
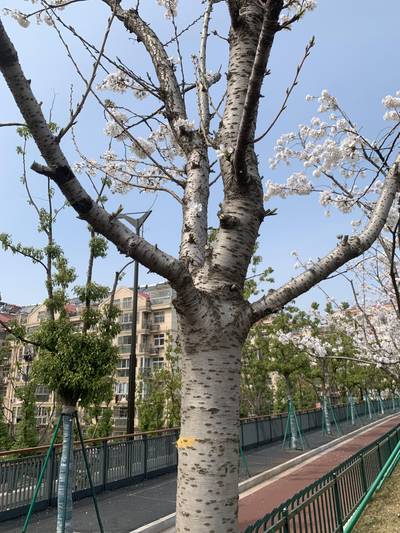

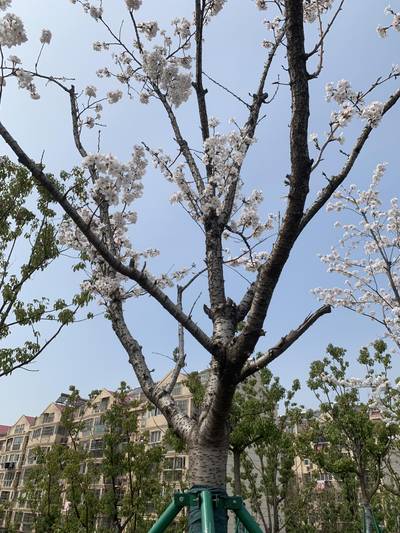
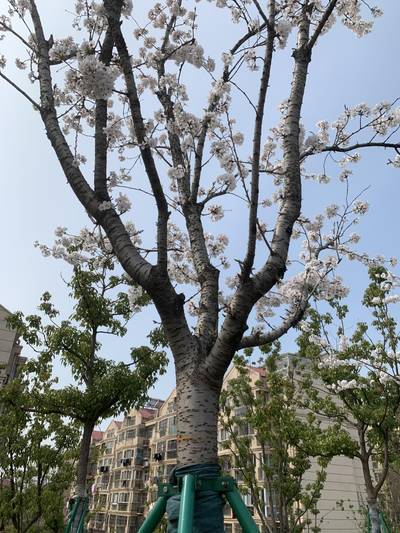
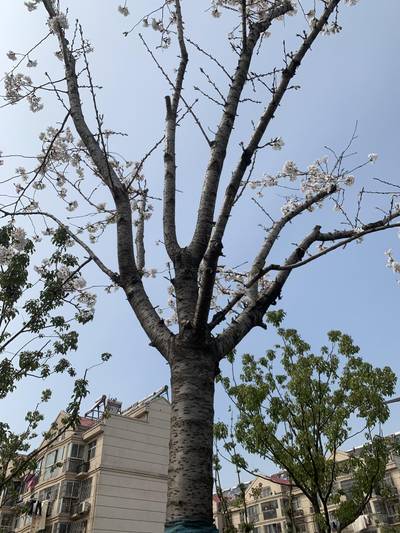


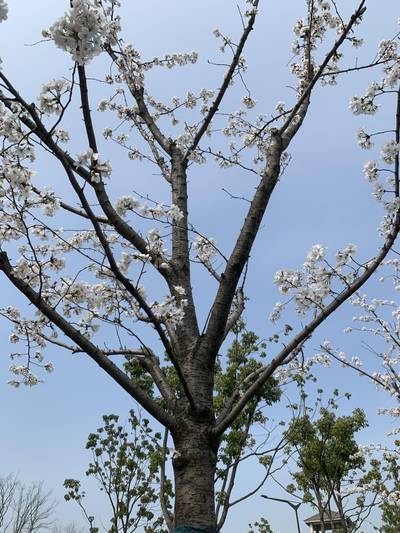
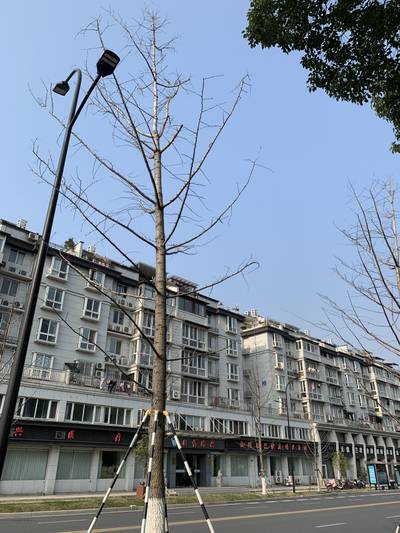
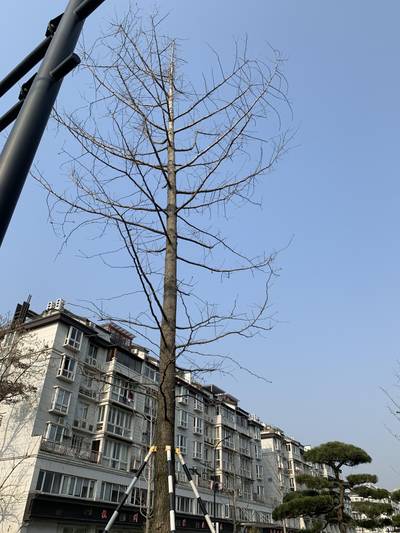
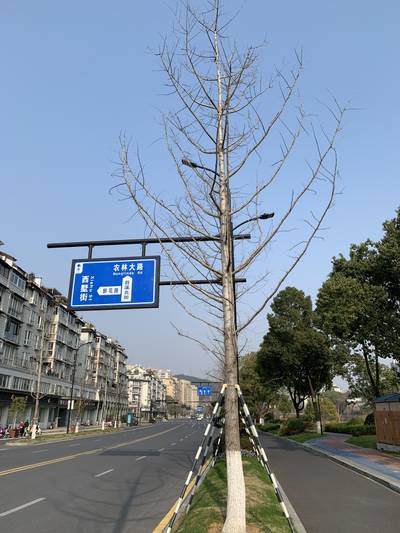
Class balance #
There are 13 annotation classes in the dataset. Find the general statistics and balances for every class in the table below. Click any row to preview images that have labels of the selected class. Sort by column to find the most rare or prevalent classes.
Class ã…¤ | Images ã…¤ | Objects ã…¤ | Count on image average | Area on image average |
---|---|---|---|---|
branch_of_flowering_cherryâž” mask | 173 | 175 | 1.01 | 16.77% |
branch_of_lagerstroemia_indicaâž” mask | 142 | 145 | 1.02 | 11.38% |
branch_of_prunus_cerasifera_f._atropurpureaâž” mask | 132 | 132 | 1 | 15.34% |
branch_of_liriodendron_chinenseâž” mask | 131 | 158 | 1.21 | 6.29% |
branch_of_zelkova_serrataâž” mask | 115 | 117 | 1.02 | 10.25% |
branch_of_magnolia_liliflora_desrâž” mask | 106 | 106 | 1 | 6.73% |
branch_of_styphnolobium_japonicumâž” mask | 102 | 103 | 1.01 | 5.31% |
branch_of_triadica_sebiferaâž” mask | 101 | 103 | 1.02 | 6.48% |
branch_of_koelreuteria_paniculataâž” mask | 100 | 100 | 1 | 9.97% |
branch_of_ginkgo_bilobaâž” mask | 100 | 106 | 1.06 | 3.93% |
Co-occurrence matrix #
Co-occurrence matrix is an extremely valuable tool that shows you the images for every pair of classes: how many images have objects of both classes at the same time. If you click any cell, you will see those images. We added the tooltip with an explanation for every cell for your convenience, just hover the mouse over a cell to preview the description.
Images #
Explore every single image in the dataset with respect to the number of annotations of each class it has. Click a row to preview selected image. Sort by any column to find anomalies and edge cases. Use horizontal scroll if the table has many columns for a large number of classes in the dataset.
Object distribution #
Interactive heatmap chart for every class with object distribution shows how many images are in the dataset with a certain number of objects of a specific class. Users can click cell and see the list of all corresponding images.
Class sizes #
The table below gives various size properties of objects for every class. Click a row to see the image with annotations of the selected class. Sort columns to find classes with the smallest or largest objects or understand the size differences between classes.
Class | Object count | Avg area | Max area | Min area | Min height | Min height | Max height | Max height | Avg height | Avg height | Min width | Min width | Max width | Max width |
---|---|---|---|---|---|---|---|---|---|---|---|---|---|---|
branch_of_flowering_cherry mask | 175 | 16.58% | 49.48% | 0.42% | 426px | 10.57% | 4032px | 100% | 3148px | 78.08% | 704px | 23.28% | 3024px | 100% |
branch_of_liriodendron_chinense mask | 158 | 5.21% | 17.88% | 0% | 47px | 1.17% | 4032px | 100% | 3065px | 76.56% | 80px | 2.65% | 3024px | 100% |
branch_of_lagerstroemia_indica mask | 145 | 11.14% | 49.52% | 1.23% | 1563px | 38.76% | 4032px | 100% | 3462px | 85.87% | 969px | 32.04% | 3024px | 100% |
branch_of_prunus_cerasifera_f._atropurpurea mask | 132 | 15.34% | 38.2% | 2.57% | 2499px | 61.98% | 4031px | 99.98% | 3521px | 87.33% | 1256px | 41.53% | 3024px | 100% |
branch_of_platanus mask | 121 | 5.72% | 19.26% | 0.52% | 944px | 23.41% | 4031px | 99.98% | 2537px | 68.49% | 485px | 12.03% | 3023px | 100% |
branch_of_zelkova_serrata mask | 117 | 10.08% | 46.04% | 0.01% | 115px | 2.85% | 4032px | 100% | 3652px | 90.58% | 150px | 4.96% | 3024px | 100% |
branch_of_magnolia_liliflora_desr mask | 106 | 6.73% | 14.29% | 1.91% | 2464px | 61.11% | 4026px | 99.85% | 3541px | 87.82% | 1166px | 38.56% | 3023px | 99.97% |
branch_of_ginkgo_biloba mask | 106 | 3.7% | 9.3% | 0.07% | 219px | 5.43% | 4032px | 100% | 3177px | 78.8% | 417px | 13.79% | 3022px | 99.93% |
branch_of_triadica_sebifera mask | 103 | 6.35% | 24.47% | 0.64% | 1072px | 26.59% | 4028px | 99.9% | 3300px | 83.46% | 893px | 23.34% | 3024px | 100% |
branch_of_styphnolobium_japonicum mask | 103 | 5.26% | 14.75% | 0.93% | 1343px | 33.31% | 4031px | 99.98% | 3120px | 77.58% | 716px | 23.68% | 3022px | 99.93% |
Spatial Heatmap #
The heatmaps below give the spatial distributions of all objects for every class. These visualizations provide insights into the most probable and rare object locations on the image. It helps analyze objects' placements in a dataset.
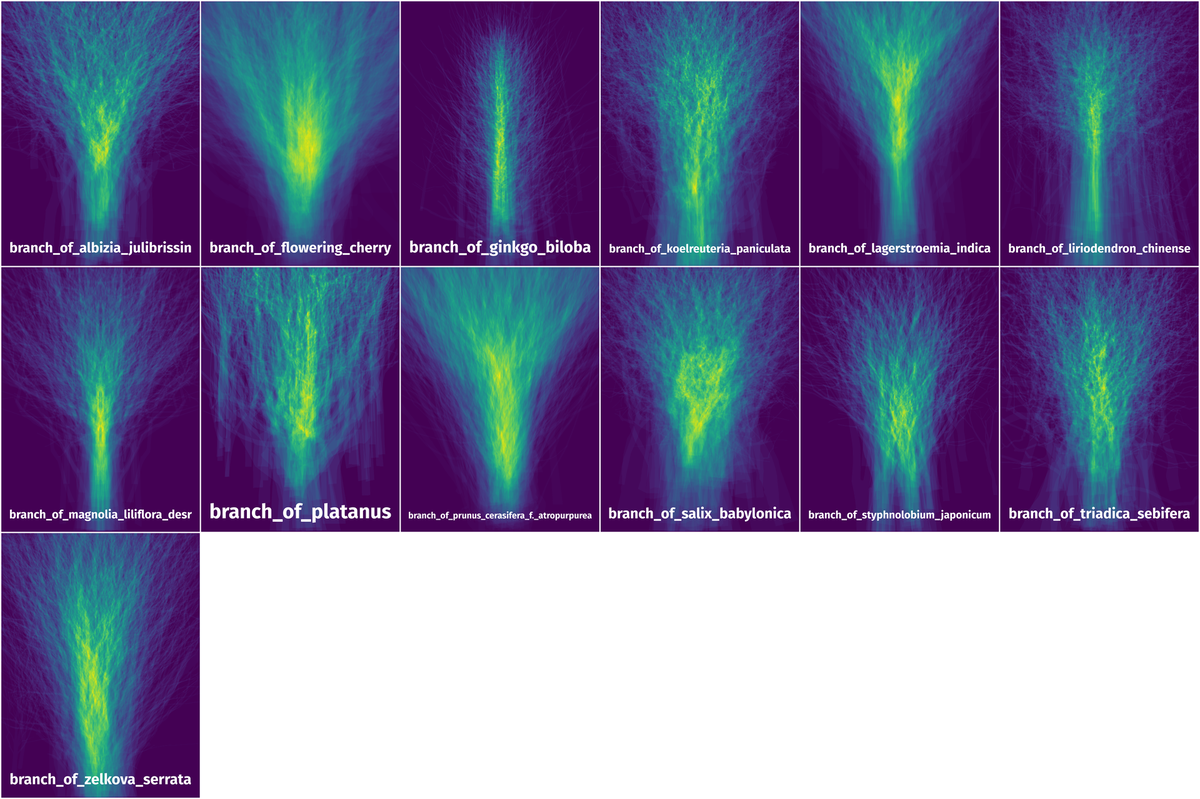
Objects #
Table contains all 1556 objects. Click a row to preview an image with annotations, and use search or pagination to navigate. Sort columns to find outliers in the dataset.
Object ID ã…¤ | Class ã…¤ | Image name click row to open | Image size height x width | Height ã…¤ | Height ã…¤ | Width ã…¤ | Width ã…¤ | Area ã…¤ |
---|---|---|---|---|---|---|---|---|
1âž” | branch_of_lagerstroemia_indica mask | Lagerstroemia indica_branch_1 (138).jpg | 4032 x 3024 | 3386px | 83.98% | 1398px | 46.23% | 6.45% |
2âž” | branch_of_styphnolobium_japonicum mask | Styphnolobium japonicum_branch_1 (101).jpg | 4032 x 3024 | 3729px | 92.49% | 2243px | 74.17% | 6.72% |
3âž” | branch_of_zelkova_serrata mask | Zelkova serrata_branch_1 (105).jpg | 4032 x 3024 | 3595px | 89.16% | 2893px | 95.67% | 10.27% |
4âž” | branch_of_triadica_sebifera mask | Triadica sebifera_branch_1 (101).jpg | 4032 x 3024 | 4010px | 99.45% | 2516px | 83.2% | 8.28% |
5âž” | branch_of_flowering_cherry mask | Flowering cherry_branch_1 (166).jpg | 4032 x 3024 | 4027px | 99.88% | 3022px | 99.93% | 22.06% |
6âž” | branch_of_styphnolobium_japonicum mask | Styphnolobium japonicum_branch_1 (100).jpg | 4032 x 3024 | 3725px | 92.39% | 3022px | 99.93% | 8.19% |
7âž” | branch_of_ginkgo_biloba mask | Ginkgo biloba_branch_1 (91).jpg | 4032 x 3024 | 3436px | 85.22% | 1260px | 41.67% | 3.33% |
8âž” | branch_of_flowering_cherry mask | Flowering cherry_branch_1 (164).jpg | 4032 x 3024 | 4025px | 99.83% | 3024px | 100% | 30.27% |
9âž” | branch_of_salix_babylonica mask | Salix babylonica_branch_1 (93).jpg | 2458 x 2206 | 2427px | 98.74% | 1915px | 86.81% | 11.96% |
10âž” | branch_of_lagerstroemia_indica mask | Lagerstroemia indica_branch_1 (133).jpg | 4032 x 3024 | 3826px | 94.89% | 2898px | 95.83% | 13.55% |
License #
Tree Dataset of Urban Street: Branch is under GNU LGPL 3.0 license.
Citation #
If you make use of the Urban Street: Branch data, please cite the following reference:
@dataset{Urban Street: Branch,
author={Tingting Yang and Suyin Zhou and Zhijie Huang and Aijun Xu and Junhua Ye and Jianxin Yin},
title={Tree Dataset of Urban Street: Branch},
year={2022},
url={https://ytt917251944.github.io/dataset_jekyll/}
}
If you are happy with Dataset Ninja and use provided visualizations and tools in your work, please cite us:
@misc{ visualization-tools-for-urban-street-branch-dataset,
title = { Visualization Tools for Urban Street: Branch Dataset },
type = { Computer Vision Tools },
author = { Dataset Ninja },
howpublished = { \url{ https://datasetninja.com/urban-street-branch } },
url = { https://datasetninja.com/urban-street-branch },
journal = { Dataset Ninja },
publisher = { Dataset Ninja },
year = { 2024 },
month = { jul },
note = { visited on 2024-07-27 },
}
Download #
Dataset Urban Street: Branch can be downloaded in Supervisely format:
As an alternative, it can be downloaded with dataset-tools package:
pip install --upgrade dataset-tools
… using following python code:
import dataset_tools as dtools
dtools.download(dataset='Urban Street: Branch', dst_dir='~/dataset-ninja/')
Make sure not to overlook the python code example available on the Supervisely Developer Portal. It will give you a clear idea of how to effortlessly work with the downloaded dataset.
The data in original format can be downloaded here.
Disclaimer #
Our gal from the legal dep told us we need to post this:
Dataset Ninja provides visualizations and statistics for some datasets that can be found online and can be downloaded by general audience. Dataset Ninja is not a dataset hosting platform and can only be used for informational purposes. The platform does not claim any rights for the original content, including images, videos, annotations and descriptions. Joint publishing is prohibited.
You take full responsibility when you use datasets presented at Dataset Ninja, as well as other information, including visualizations and statistics we provide. You are in charge of compliance with any dataset license and all other permissions. You are required to navigate datasets homepage and make sure that you can use it. In case of any questions, get in touch with us at hello@datasetninja.com.